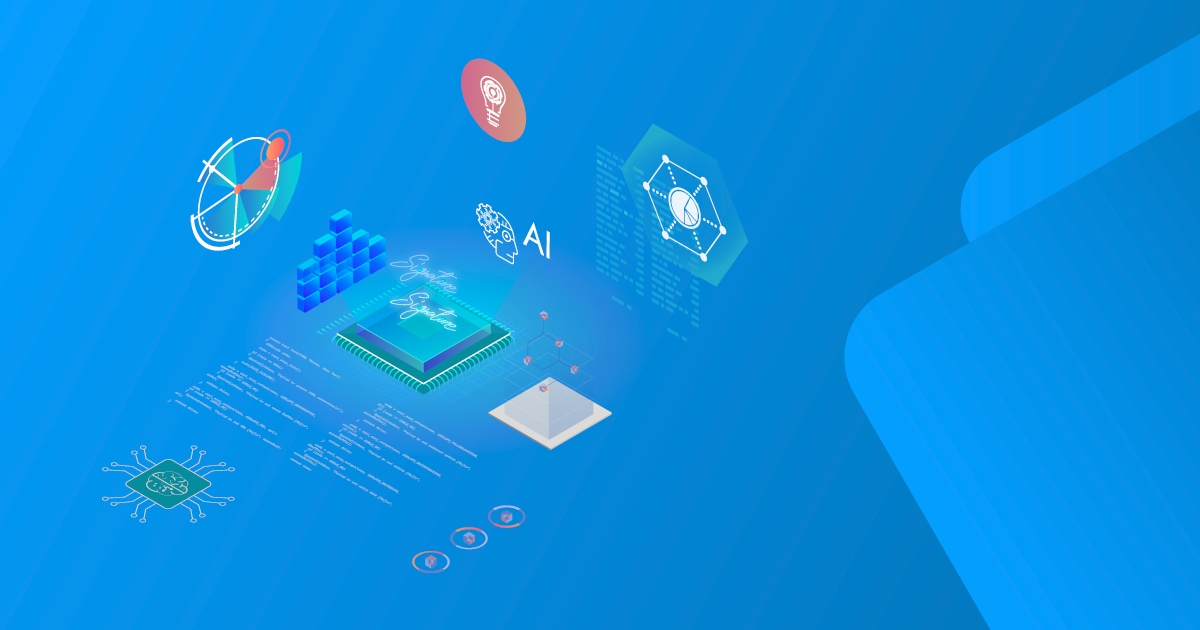
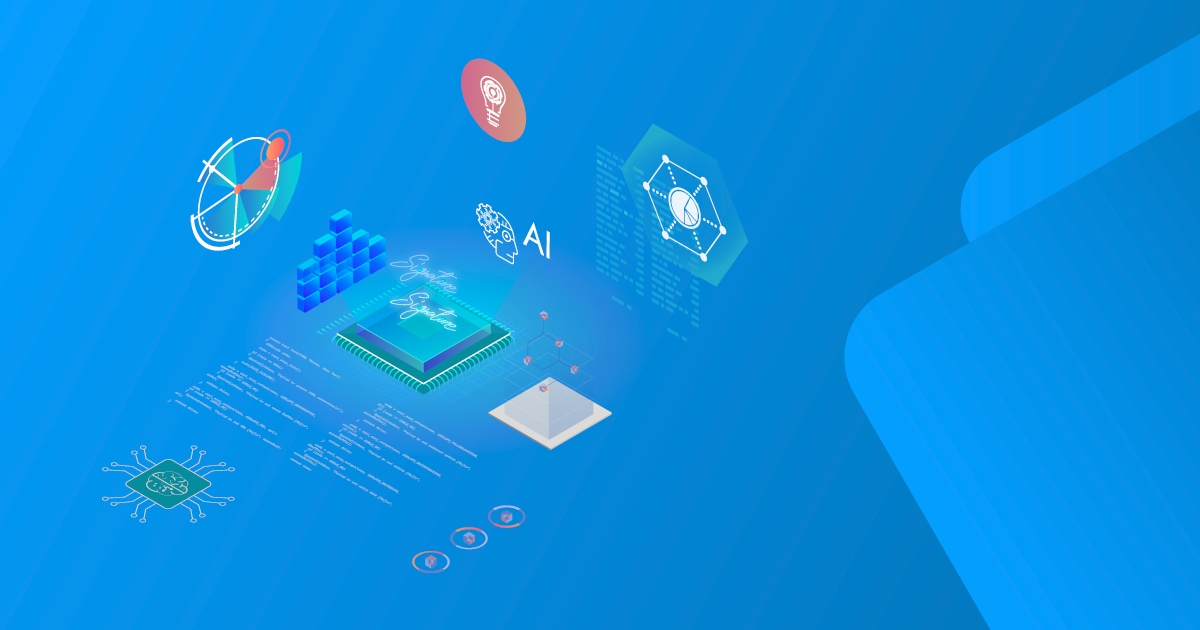
Transforming Signature Verification with Machine Learning
In today's digital era, the need for accurate and efficient signature verification is paramount across industries. Traditional manual processes are time-consuming and prone to error. However, the advent of machine learning and automatic signature verification has revolutionized this landscape, offering significant advancements in efficiency and precision.
This article explores the transformative impact of machine learning on signature verification, highlights the key benefits it brings, and discusses its widespread adoption beyond the financial sector, such as in insurance, telecommunications, and more.
Transforming Signature Verification with Machine Learning Vs. Manual Handling
Unprecedented Speed and Efficiency:
Traditional manual verification relies on visual inspection, which can be difficult and time-consuming. Machine learning-powered automatic signature verification systems leverage advanced algorithms and techniques to swiftly analyze and compare signatures against reference samples. This accelerates the verification process, expediting document processing and transaction workflows. By automating the process, machine learning minimizes human errors caused by fatigue or oversight, leading to significantly improved speed and efficiency.
Unmatched Accuracy and Consistency
Manual verification processes are subjective and prone to inconsistencies. Machine learning algorithms excel in signature verification by analyzing millions of parameters. These algorithms rely on objective measurements, ensuring higher accuracy and consistency. By eliminating the limitations of human judgment, machine learning-powered systems minimize errors caused by variations in perception, subjective interpretations, or biases, leading to unparalleled accuracy and reliability.
Advanced Fraud Detection and Prevention
Detecting fraudulent signatures is a critical aspect of verification processes. Machine learning algorithms have revolutionized fraud detection by being able to identify subtle anomalies and patterns that may indicate forgery. These algorithms learn from vast amounts of training data, enabling them to detect forged signatures, and even signature replicas. By enhancing security measures, machine learning-powered systems protect organizations from potential losses and bolster trust in transactions.
Scalability and Adaptability
As the volume of digital transactions and document processing continues to grow, scalability becomes essential. Machine learning-based automatic signature verification systems offer unparalleled scalability, effortlessly handling a large number of signatures without compromising accuracy. Moreover, these systems can adapt to evolving signature styles, cultural differences, and individual variations. They are not limited by specific document formats or languages, making them highly versatile in accommodating the needs of different industries.
The Pervasive Adoption of Machine Learning in Signature Verification
Machine learning technologies have paved the way for the widespread adoption of automatic signature verification across diverse sectors. Beyond the financial industry, machine learning-powered systems have found applications in insurance, telecommunications, healthcare, government, and human resources.
In the insurance sector, automatic verification expedites policy issuance and claims processing, enabling faster responses and improved fraud prevention. Telecommunications companies benefit from streamlined contract management and enhanced compliance. In healthcare, machine learning-based verification ensures accurate medical records and patient safety. Government agencies leverage automation to enhance legal document authenticity and streamline administrative processes. Human resources departments can optimize onboarding, contract management, and personnel administration through machine learning-powered verification.
Conclusion
Machine learning has transformed the landscape of signature verification, offering unprecedented accuracy, speed, and scalability. By harnessing advanced algorithms and image processing techniques, machine learning-powered systems have revolutionized the way signatures are verified, significantly reducing manual errors.
As machine learning technology continues to advance, we can expect even greater enhancements in automatic signature verification, empowering organizations to streamline operations, enhance customer experiences, and ensure the integrity of critical transactions. Embracing this innovative technology is a pivotal step towards a future where manual errors are dramatically reduced, and trust and efficiency thrive in the digital landscape.
You May Also Like
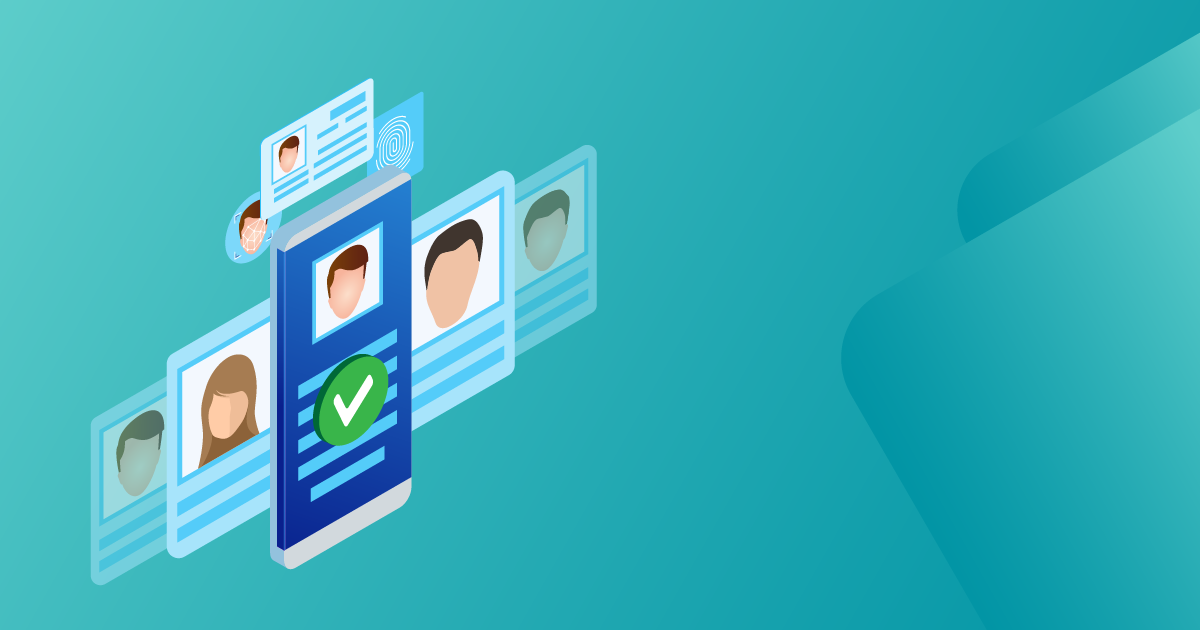
From Ink to Clicks: Unraveling the eKYC Evolution
Identity verification has undergone a monumental digital revolution since the introduction of electronic Know Your Customer (eKYC) solutions. Delve into this article to uncover how this innovation has redefined operations and laid the groundwork for a promising trajectory!
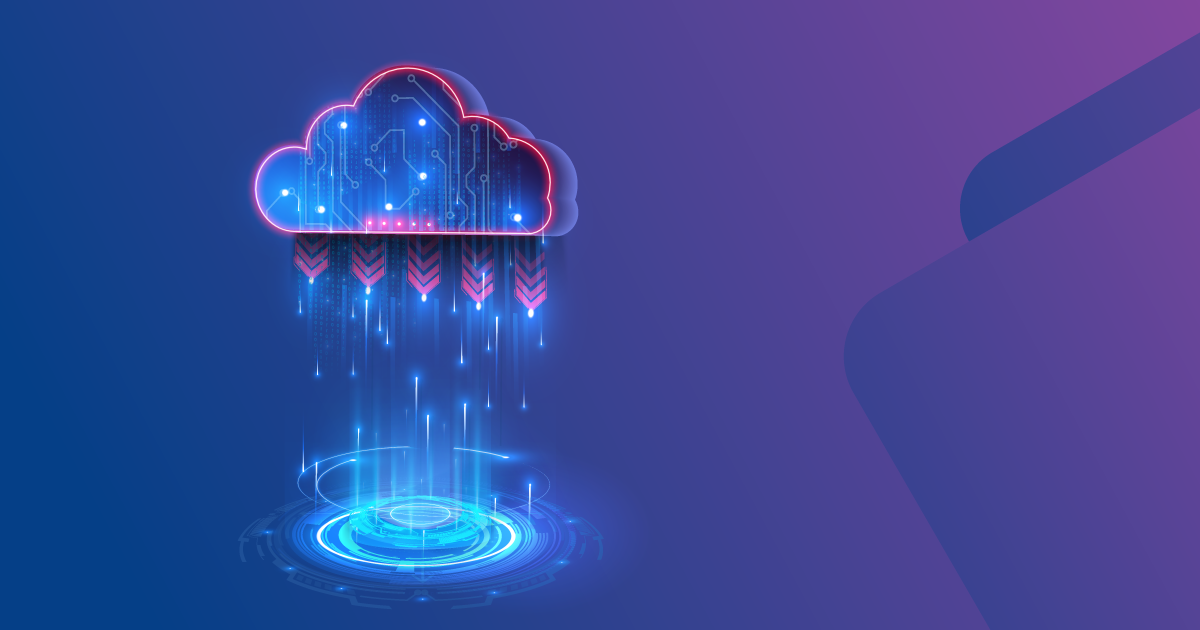
5 Challenges Cloud-native Infrastructure Can Help You Overcome
As the global landscape rapidly embraces automation and cutting-edge technologies, cloud-native infrastructure emerges as the optimal solution for organizations aiming to effortlessly scale and optimize their operations. But what are the barriers that cloud-native infrastructure has completely dismantled? Discover them by diving into this article!
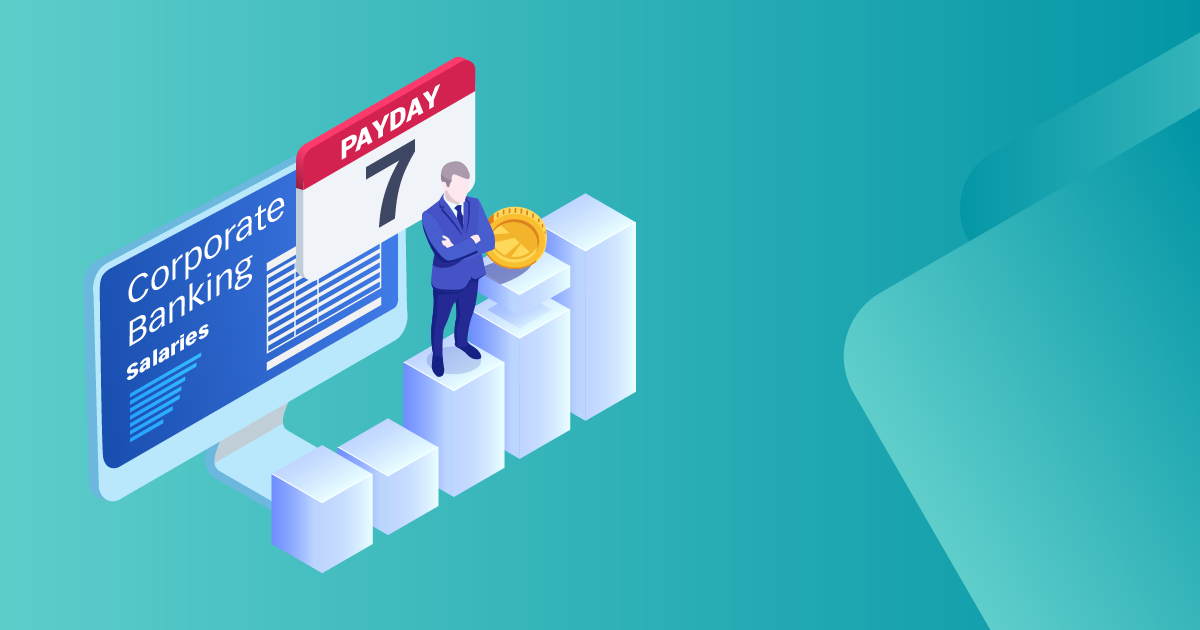
8 Ways Corporate Banking Redefines Salary Payments
Within the array of benefits offered by corporate banking, salary payments stand out as a cornerstone for businesses, streamlining manual-intensive tasks, boosting convenience and paving the way for true digital transformation. Delve into the article to explore the innovative facets of corporate banking that reshape salary payments and defy conventional methods.